Exploring Predictive Analytical Models for Businesses
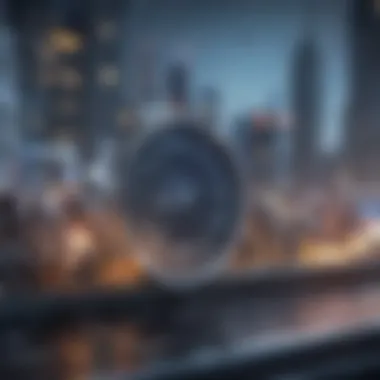
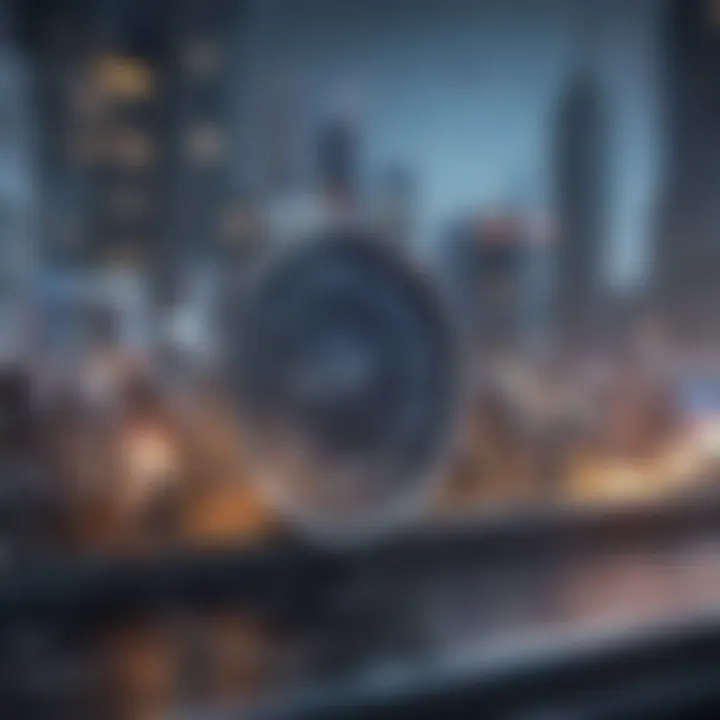
Intro
Predictive analytics isn’t just a buzzword thrown around in boardrooms; it’s the heartbeat of contemporary data-driven decision-making. In an increasingly complex and fast-paced world, businesses are constantly bombarded with a tsunami of data. The real challenge lies in deciphering this mass of numbers and transforming it into actionable insights. Imagine trying to find a needle in a haystack, where the haystack is an ocean of data, and the needle represents crucial insights that could steer a company towards profitability.
Through the perspective of predictive analytical models, organizations can better navigate the uncertainties of their respective landscapes. These models not only assist in forecasting market trends but also in understanding customer behaviors, thus empowering businesses to make informed decisions.
In this explorative journey, we will unearth the fundamentals of predictive analytics, focusing on the techniques, limitations, risks, and future outlook of these models. Each piece of this narrative works to demystify the complexities involved and demonstrates the practicality and necessity of predictive analytics across various sectors, particularly finance.
Investment Terminology and Concepts
Before delving into the nitty-gritty of predictive models, it is essential to ground ourselves in the fundamental investment terms and concepts that lay the groundwork for effective analysis.
Key Investment Terms Explained
Understanding the language of finance is as crucial as grasping predictive analytics itself. Here are some key terms that frequently surface in discussions about predictive models:
- Predictive Model: A mathematical tool designed to make predictions about future outcomes based on historical data.
- Regression Analysis: A statistical method used to assess the relationships between various factors, often used in developing predictive models.
- Forecasting: The process of estimating future events or conditions based on historical data.
- Machine Learning: A subset of artificial intelligence that improves model predictions as more data becomes available.
Understanding Risk and Reward
Every investment comes with its share of risks and potential rewards. In the realm of predictive analytics, the balance of these two elements can make or break a strategy.
Consider the following:
- Risk Tolerance: Knowing how much risk an organization can withstand is crucial. Predictive models can assess potential risks associated with different decisions, helping businesses avoid pitfalls.
- Reward Potential: With every risk, there is the possibility of reward. Predictive models provide insights that can boost profitability and efficiency, steering companies toward lucrative ventures.
"Risk management is about understanding the downside." - Unknown
This adage underscores the significance of solid predictive frameworks in sculpting a balanced investment strategy, reminding investors and analysts that being cautious does not exclude the pursuit of opportunity.
In the subsequent sections, we will delve deeper into the specifics of predictive analytical models, exploring how they are developed, evaluated, and applied in various industries, showcasing their impact on business strategy and outcomes.
Prolusion to Predictive Analytical Models
In an age where data reigns supreme, understanding predictive analytical models is akin to having a crystal ball in the modern business landscape. These models harness statistical techniques and machine learning algorithms to forecast future events based on historical and current data. As organizations strive for an edge in decision-making amidst relentless competition, leveraging these models becomes not just beneficial but essential.
Predictive analytics operates at the intersection of data and strategy, enabling businesses to anticipate trends, improve customer experiences, and optimize resources. The benefits are manifold:
- Enhanced Decision-Making: By providing insightful forecasts, these models help stakeholders make informed decisions rather than relying on gut feelings or outdated information.
- Risk Management: Identifying potential pitfalls ahead of time allows organizations to mitigate risks effectively, preserving resources and reputation.
- Performance Optimization: With the right predictive techniques, businesses can streamline operations, enhance productivity, and subsequently increase profitability.
However, there's more than meets the eye when it comes to predictive analytical models. The intricacies involved in crafting these models require a thorough understanding of both the data they utilize and the specific industry context. Missteps during the data preparation phase can lead to invalid conclusions. Likewise, the interpretation of results demands an analytical mindset and a grasp of underlying principles.
Therefore, diving into predictive analytical models isn’t merely an academic exercise. It’s a complex journey laden with opportunities and challenges, shaping the frontline of decision-making in various sectors. As this exploration unfolds, we’ll delve into the definition and scope of predictive analytics, tracing its historical roots and outlining its evolution in contemporary applications.
Understanding Predictive Analytics
Predictive analytics plays a critical role in the modern data landscape. With the power to forecast outcomes and guide decision-making, understanding this field becomes essential for investors, financial advisors, analysts, educators, and students alike. It harnesses historical data to make informed predictions about future events. This can provide stakeholders with a significant edge, particularly in today’s fast-paced environment where every insight counts.
The importance of predictive analytics manifests in several key areas:
- Informed Decision-Making: Organizations leverage these models to base their strategies on data rather than intuition alone. This leads to more successful outcomes.
- Risk Management: By identifying potential challenges ahead of time, businesses can implement strategies to mitigate risks, making predictive analytics invaluable in financial environments.
- Resource Optimization: Efficient allocation of resources can be achieved through precise forecasting, enhancing overall productivity and profitability.
Core Principles
The core principles of predictive analytics rest on understanding data, statistical methodologies, and domain knowledge. The convergence of these elements leads to actionable insights. At the heart of predictive modeling is the concept that patterns in past data can shed light on future occurrences.
Key principles include:
- Data Relevance: Ensuring the datasets acquired for analysis are pertinent to the predictive question at hand.
- Statistical Modeling: Utilizing mathematical frameworks and algorithms for developing reliable models.
- Continuous Monitoring and Maintenance: As new data flow in, models require updates to maintain their accuracy and relevance.
Each principle intertwines, creating a robust framework for generating accurate forecasts.
Types of Predictive Models
Predictive models are more than just algorithms; they're tailored approaches designed to meet specific forecasting needs. In this section, we will explore three main types of predictive models: regression models, classification models, and time series models. Each has its own nuances, applications, and distinctive features contributing to the broader landscape of predictive analytics.
Regression Models
Regression models are a cornerstone of predictive analytics, enabling analysts to understand relationships between variables. By predicting a dependent variable based on one or more independent variables, they provide clarity in forecasts. A key characteristic of regression models is their focus on estimating outcomes, making them particularly beneficial in scenarios such as financial forecasting and real estate valuation.
Unique features of regression models include:
- Interpretability: Results can often be explained in straightforward terms, allowing stakeholders to grasp the implications clearly.
- Flexibility: There are various types of regression models, from linear to polynomial, catering to different data distributions.
However, one must be cautious of potential assumptions the model relies upon, which could lead to inaccurate predictions if not met.
Classification Models
Classification models stand out by putting data into specific categories. They are widely used in various sectors, especially in finance where assigning credit risk levels is crucial. The defining characteristic of classification models is their capability to sort data into discrete class labels, which helps in making binary or multi-class decisions.
Key features include:
- Efficiency in Handling Large Datasets: These models can process significant volumes of data, making them suitable for industries flooded with information.
- Robust Evaluation Metrics: They provide a range of metrics such as precision, recall, and F1-score to assess model performance.
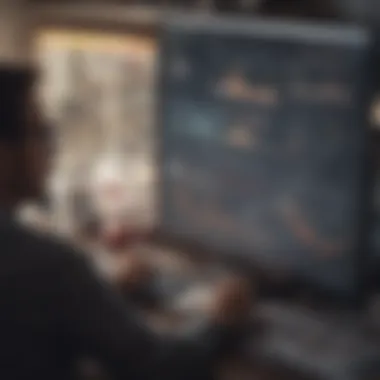
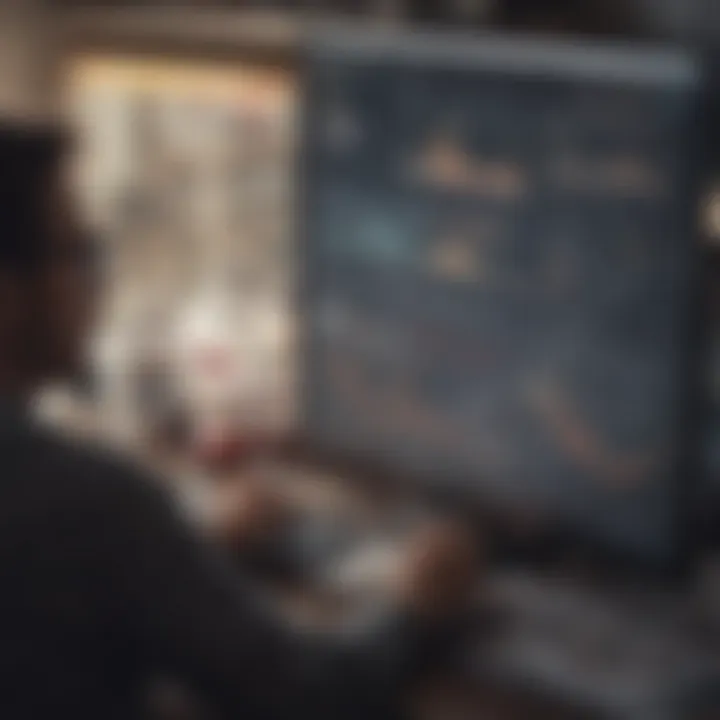
The downside? Classification models sometimes oversimplify complex problems, losing the nuances of the data.
Time Series Models
Time series models are designed explicitly for forecasting based on data collected over time. They capitalize on temporal trends and patterns, making them ideal for stock price predictions or economic forecasts. Their key characteristic lies in accurately capturing and utilizing time-dependent information.
Notable aspects include:
- Trend Analysis: Time series models excel in identifying patterns over time, which can forecast future values effectively.
- Autoregressive Features: Many models rely on previous time points, allowing them to adjust in response to fluctuations.
On the flip side, time series models can be sensitive to outliers, which may skew predictions if not managed properly.
Understanding the nuances of each type of predictive model enhances the reader's capacity to harness these tools effectively. The art of selection becomes crucial when deciding which model to apply, as each scenario may require a different approach.
Data Collection and Preparation
Data collection and preparation serve as the bedrock of predictive analytical models. All the statistical magic and algorithmic prowess in the world won’t avail you much if the foundation is shaky. The significance of this phase cannot be overstated; it dictates the viability and reliability of the insights you hope to extract. By meticulously gathering and preparing data, analysts can ensure that predictive models are built on precise, relevant information, yielding more accurate predictions that can genuinely influence decision-making.
Identifying Relevant Data Sources
When it comes to harnessing the power of predictive analytics, the first step is recognizing where to gather your data from. This is like finding your way through a maze—one wrong turn, and you might end up with information that’s outdated or irrelevant. Sources can be diverse, including:
- Internal Data: Think of customer databases, sales logs, and operational records. These are the nuggets of gold that organizations usually overlook. They can provide rich insights into behaviors and patterns.
- External Data: This might include market reports, social media analytics, or demographic data from platforms like Facebook. When pinpointing these sources, consider their credibility and relevance. It's crucial that the information aligns with the questions you’re trying to answer.
- Open Data: Many governments and organizations publish data freely, which can be a boon for analysts. The trick here is sifting through it to identify what’s useful.
Understanding which sources to tap into means considering both the data's context and your project's objectives. Without alignment here, you might end up comparing apples to oranges.
Data Cleaning Techniques
Once you've rounded up your data, the next phase kicks in—cleaning it up. Think of data cleaning as decluttering a messy room; you need to toss out the junk to appreciate the good stuff.
Common data cleaning techniques include:
- Removing Duplicates: Nobody wants to see double. Duplicate records can mislead models into thinking that certain patterns are more substantial than they actually are.
- Addressing Missing Values: You might encounter gaps in your data. Sometimes it’s okay to fill in these blanks with averages or medians, while other times, you’re better off removing the affected records. This choice depends on the extent and implications of the missing data.
- Filtering Irrelevant Information: Not every column or data point will matter. For instance, piecing together a customer’s purchase history might not require knowing their favorite color. Trimming the fat helps in focusing the model on what’s truly relevant.
Here, attention to detail is essential. Skipping this step can skew the model's output, leading to conclusions that are as useful as a screen door on a submarine.
Feature Selection and Engineering
Feature selection and engineering are where the fun begins. This phase involves distilling and enhancing your data into attributes that best describe the phenomenon you’re examining—like selecting the best ingredients for a gourmet dish.
- Feature Selection: This is about identifying which data features (variables) contribute the most to your model's predictive power. You might use techniques such as recursive feature elimination or feature importance ranking to cut the noise and focus on what truly matters.
- Feature Engineering: This goes a step further. Say you have a timestamp; you can engineer features that may reveal hidden patterns such as the time of day, weekday, or even seasonality. Crafting such features can vastly improve the model’s performance, much like fine-tuning a musical instrument can enhance the overall sound.
The interplay between feature selection and engineering often determines how well a model performs. Spending time here is not just advisable; it’s indispensable.
"Data is not just a collection of numbers; it is the lifeblood of predictive analytical models."
In summary, data collection and preparation lay the crucial groundwork for predictive analytics. The effort spent in identifying data sources, cleaning the data, and selecting or engineering features pays off tremendously when developing robust predictive models.
Model Development Process
The model development process stands as a cornerstone in the landscape of predictive analytics. It is in this phase that raw ideas begin to take form, morphing into robust predictive models that guide data-driven decisions. Understanding this process, from selecting algorithms to fine-tuning models, is paramount for anyone venturing into the realm of predictive analytics.
Developing a predictive model is not merely about finding patterns in data; it’s also about ensuring those patterns translate into reliable forecasts. Each stage of the process—selecting the right algorithm, training the model, and refining it through parameter tuning—involves careful consideration of the data and the ultimate objectives of the analysis.
Choosing the Right Algorithm
Selecting the appropriate algorithm is akin to choosing the right tool for a job. The choice significantly impacts the model's efficiency and its predictive power. Different algorithms have different strengths and weaknesses, and they behave uniquely based on the nature of the data at hand.
- Understanding the goal: Is the objective to classify data points, predict continuous outcomes, or analyze time-series trends? For instance, if you're working within a financial context and need to categorize clients based on credit risk, classification algorithms like logistic regression or decision trees may be suitable.
- Algorithm Characteristics: Some algorithms excel with large datasets, others with small, dense datasets. For example, support vector machines might work well for high-dimensional data, while basic linear regression could suffice for smaller datasets with a clear linear relationship.
- Experimentation and Testing: Often, the right algorithm is not apparent from the outset. Conducting preliminary analyses can yield insights. Rather than sticking with a single choice, it would be prudent to experiment with several algorithms during the development phase to gauge their performance against your objectives.
Model Training Techniques
Training a model is like a nurturing process—feeding it the right inputs so it can learn effectively. The quality of training data, the method of training, and the duration all shape the learning efficacy of the model.
One must consider:
- Data Splitting: It is common practice to split the dataset into training and testing subsets. This approach ensures that the model does not just memorize the data but learns to generalize beyond it. A typical split could be 70% training and 30% testing.
- Iterative Training: The model must be trained repeatedly, refining it to improve performance through consistent feedback loops. Each iteration allows the model to adjust its predictions based on its previous errors, gradually honing its ability to make accurate predictions.
- Incorporating Domain Knowledge: Integrating insights from domain experts can enhance the training process. Their input might reveal nuances within the data that can influence the learning process, providing guidance in feature selection or interpreting results.
Parameter Tuning
Parameter tuning is a critical step in model development that can not be overlooked. It involves adjusting model settings to boost accuracy. Thoughtful tuning enhances the model's ability to make accurate predictions while avoiding both overfitting and underfitting.
Key considerations include:
- Grid Search and Random Search: These techniques automate the search for optimal parameters. Grid search evaluates a range of parameter combinations, while random search samples a selection from the parameter space. Both can efficiently identify settings that yield peak performance.
- Validation: Using techniques like k-fold cross-validation during tuning helps gauge how the model performs with varying data subsets, providing a more comprehensive view of potential performance.
- Monitoring Changes: It’s essential to closely monitor how parameter adjustments impact predictions. Graphing the model's accuracy against parameter values can help visualize relationships and guide decisions.
The model development process is a blend of science and art, requiring careful thought, a willingness to innovate, and a commitment to continual learning.
In summary, the model development process plays a pivotal role in predictive analytics. From choosing the right algorithm to refining model parameters, each step is crucial for creating a reliable, effective predictive model. This foundational process enables businesses and organizations to leverage data for informed decision-making, driving success in an increasingly competitive landscape.
Evaluation of Predictive Models
Evaluating predictive models is akin to holding a magnifying glass to the intricate workings of data-driven systems. This process doesn't just validate the reliability of models but also ensures that the decisions made from them are sound and informed. Every model has its strengths and weaknesses, and being able to identify these characteristics can mean the difference between making decisions based on solid ground or walking on thin ice.
Some key elements to consider when evaluating predictive models include the metrics used for assessment, cross-validation techniques, and an overall understanding of the model's performance in real-world scenarios. A nail-on-the-head evaluation can provide valuable insights into the model’s effectiveness, its adaptability to new data, and its potential limitations. Evaluating models also helps reinforce principles related to data quality and the appropriateness of chosen algorithms.
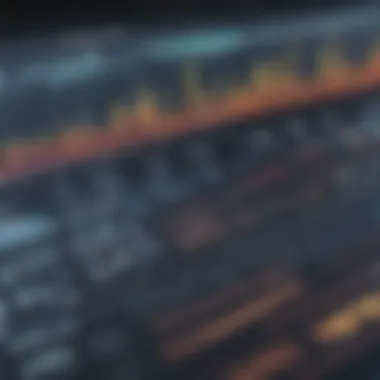
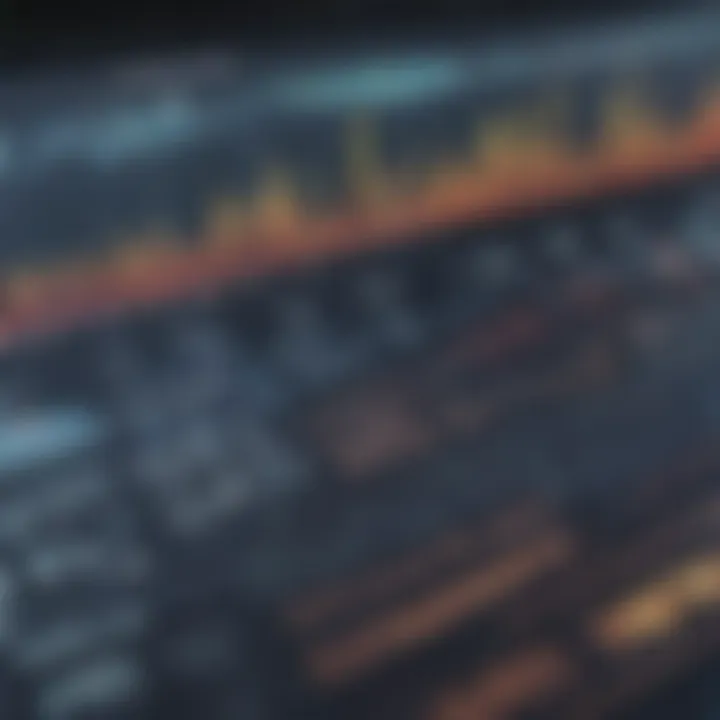
Metrics for Model Assessment
Accuracy
Accuracy represents the proportion of true results (both true positives and true negatives) among the total number of cases examined. It is often seen as a straightforward measure for performance. This metric offers a clear picture at first glance, making it a popular choice in various analytical settings. Having a model with high accuracy is generally sought after since it suggests that the model can correctly predict outcomes.
However, it’s essential to recognize that accuracy can sometimes be misleading, especially in scenarios with class imbalance. For instance, in a medical diagnosis model where 95% of patients are healthy, a model that simply predicts every patient as healthy might still achieve 95% accuracy, yet it completely fails to identify the ill.
Key Characteristics of Accuracy:
- Provides a general performance overview.
- Useful where classes are balanced.
Unique Features of Accuracy:
- Simple to compute and interpret.
- May hide deeper issues in model performance.
Precision and Recall
Precision and recall are crucial for prioritizing the quality of positive predictions. Precision is the fraction of true positive predictions out of all positive predictions made, while recall is the fraction of true positives that were correctly identified by the model. These metrics are especially beneficial in situations where correctly identifying positive cases is more critical than identifying negative ones.
When it comes to cost-sensitive applications, such as fraud detection or disease screening, having high precision ensures that the number of false positives is minimized. Recall on the other hand emphasizes capturing every actual positive, which might be slightly different. If a model has a high recall but lower precision, it might be producing many false alarms, which can lead decision-makers to act on signals that aren't trustworthy.
Key Characteristics of Precision and Recall:
- Focus on quality rather than quantity of predictions.
- Allows for a nuanced understanding of model performance in skewed datasets.
Unique Features of Precision and Recall:
- Trade-off between precision and recall can be visualized using F1 Score.
- Vital metric in contexts where the cost of false positives is high.
ROC Curves
ROC (Receiver Operating Characteristic) curves provide a graphical representation of a model's diagnostic ability as its discrimination threshold is varied. In essence, ROC curves plot the true positive rate against the false positive rate, helping visualize how well a model distinguishes between classes.
This tool is notably advantageous because it provides insights across a spectrum of thresholds rather than being tied to a single operating point. The area under the ROC curve (AUC) indicates the model’s ability to discriminate between the positive and negative classes, with a higher value suggesting better performance.
Key Characteristics of ROC Curves:
- Offers a threshold-independent evaluation of model performance.
- Easily interpretable and visually impactful.
Unique Features of ROC Curves:
- Highlights trade-offs between sensitivity and specificity.
- Useful in comparing multiple models simultaneously.
Cross-Validation Techniques
Cross-validation is a critical step in robust model evaluation, allowing practitioners to assess how a model performs on unseen data. This technique involves partitioning the original dataset into subsets to provide a more reliable understanding of a model's potential effectiveness. Common methods include k-fold cross-validation, which divides the dataset into 'k' parts and iteratively trains models on k-1 parts while testing on the remaining piece.
In short, by using cross-validation, one can be more confident that their model will hold up when it encounters new data. With every fold, one gets a little better at knowing which models are glossing over important details and which are genuinely effective. In the end, the evaluation of predictive models is an essential part of the predictive analytics workflow; a thorough assessment paves the way for more informed decisions and deeper understanding of both the data and the model's implications.
Applications Across Industries
The significance of predictive analytical models in today’s world cannot be overstated. These frameworks have gained traction across various sectors, serving as pivotal tools that empower organizations to make data-driven decisions. As the demand for efficiency and precision increases, the application of predictive analytics becomes that much more vital.
By leveraging these models, businesses can better predict outcomes, optimize processes, and craft tailored strategies that directly correspond to their unique contexts and objectives. Each industry faces its own particular challenges, yet the benefits of applying predictive analytics are apparent. Here’s an exploration of how this approach is resonating across four major fields.
Finance and Banking
In the finance sector, predictive analytical models play a critical role in risk management and investment strategies. Banks utilize these models to forecast loan defaults or assess the creditworthiness of potential borrowers.
- The ability to analyze historical data can guide financial institutions in recognizing patterns.
- For example, if a bank notices an uptick in late payments among a demographic group, it may adjust its lending policies accordingly.
Beyond traditional lending, investment firms employ predictive analytics to optimize portfolios, predicting which assets are likely to perform well. The intricacies of stock price movements are dissected using various machine learning algorithms, which can lead to more informed trading decisions.
Healthcare Predictions
Predictive analytics in healthcare is transformative, as it allows for anticipating patient outcomes and enhancing operational efficiencies. Healthcare institutions harness data to identify at-risk patients, enabling timely interventions.
For instance, by analyzing hospital records, a predictive model might reveal that patients with specific demographics or pre-existing conditions have a higher likelihood of readmission within thirty days of discharge.
Key benefits include:
- Reduced healthcare costs: By preventing avoidable readmissions.
- Improved patient outcomes, ensuring timely and personalized care.
- Streamlined resource allocation, allowing hospitals to manage staffing and equipment based on anticipated needs.
Marketing Strategies
In marketing, the integration of predictive analytics allows companies to anticipate customer behavior. Through analyzing demographic data, purchasing patterns, and sentiment analyses, businesses can craft targeted campaigns that resonate with their audiences.
Consider the way a firm like Amazon or Netflix provides recommendations.
- Customer segmentation helps marketers identify specific group needs.
- Churn prediction models keep businesses a step ahead of potential customer losses, informing retention strategies.
In essence, these models assist marketers in being proactive rather than reactive, fostering customer loyalty and enhancing the overall customer experience.
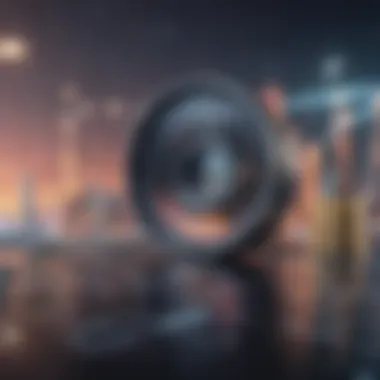
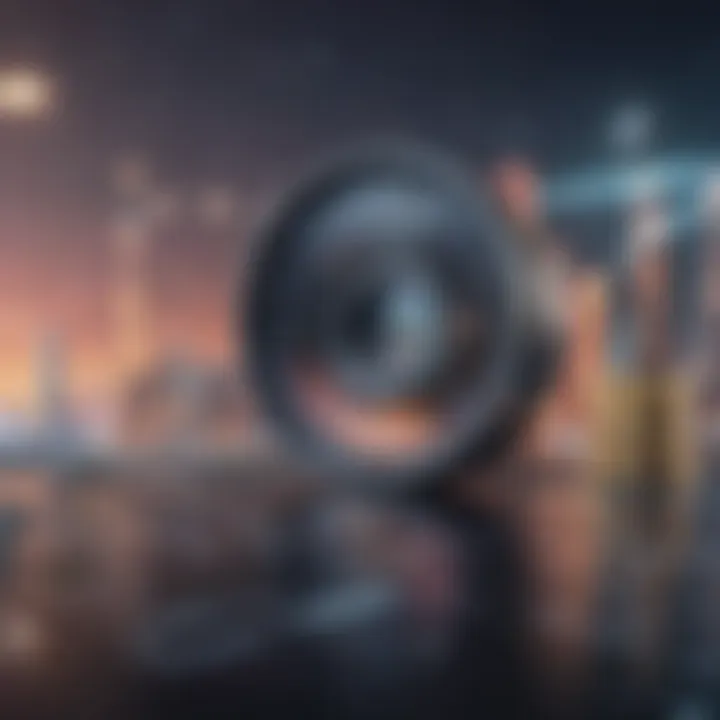
Manufacturing and Supply Chain
The applications in manufacturing and supply chain management are numerous, focusing on predictive maintenance and demand forecasting.
Organizations utilize predictive models to determine the likelihood of equipment failure, allowing for scheduled maintenance before actual breakdowns occur. This not only saves costs but also minimizes production downtime.
Furthermore, by analyzing data on consumer demand trends, companies can streamline inventory management, ensuring they have the right products on hand without overstocking.
In summary, predictive analytical models wield great influence across sectors. With the power to foresee challenges and capitalize on unseen opportunities, these models are becoming staples in decision-making processes across the board.
Challenges and Limitations
Navigating the realm of predictive analytical models isn't a walk in the park. While these models shine a light on potential outcomes, they also come with their fair share of obstacles. Understanding these challenges is crucial for anyone diving into the use of predictive analytics in business or research. Addressing limitations not only enhances model performance but also builds trust in the outputs generated, particularly among investors and stakeholders.
Data Quality Issues
Data quality stands as a cornerstone of any successful predictive model. Simply put, if the data is flawed, the outputs will surely follow suit. Raw data can harbor various inconsistencies, including missing values, inaccuracies, and irrelevant information, often leading to a snowball effect in the conclusions drawn.
- Missing Values: When data points are absent, it can skew the analysis. Dealing with missing information is crucial. Techniques such as imputation or simply removing incomplete records demand careful thought.
- Inaccurate Data: Erroneous entries can stem from human error or outdated information. If a financial model relies on incorrect historical data, the forecast it produces may lead to misguided investment decisions.
- Relevance of Data Sources: Not all data is created equal. It's essential to evaluate if the data collected aligns with the objective of the predictive analytics task. Gathering data from reputable sources mitigates risks associated with poor-quality data.
Ultimately, enhancing data quality means not only better outcomes but also fostering confidence among users of predictive models.
Overfitting and Underfitting
When developing predictive models, one must walk a fine line between overfitting and underfitting.
- Overfitting occurs when a model learns the training data too well, capturing noise rather than the underlying trend. This results in a model that performs brilliantly on historical data but crumbles in real-world applications. Think of it like memorizing answers for an exam; knowing the answers doesn't equate to understanding the subject.
- Underfitting, on the other hand, happens when a model is too simplistic. It fails to capture the underlying trend, leading to poor predictive power. Striking a balance between these two extremes is critical. Common strategies to avoid these pitfalls include performing feature selection judiciously and using techniques like cross-validation to gauge your model’s performance.
Achieving a well-rounded model contributes to reliable predictions helping stakeholders make informed decisions.
Ethical Considerations
In today's world, ethical standards are pivotal, and predictive models are not exempt from scrutiny. One major area of concern is bias in data. An algorithm trained on biased data can perpetuate and even exacerbate inequality.
- Bias in Data: If historical data reflects a skewed perspective or discrimination based on gender, race, or socioeconomic factors, the model then risks producing similarly biased outcomes. This not only harms those disadvantaged groups but may also lead to reputational damage for organizations relying on these predictions.
- Privacy Concerns: The collection of user data raises significant ethical issues. Companies must tread carefully, ensuring compliance with regulations such as GDPR and maintaining transparency with data usage.
- Responsibility of Outcomes: With the output of predictive analytics impacting real lives, it's imperative for organizations to establish a framework of accountability in their model deployments. Stakeholders need clarity on how decisions are made and a guarantee that ethical standards are upheld.
As the field progresses, upholding ethical considerations is critical, not just for compliance but for maintaining trust among those who are being analyzed.
"In the age of data, predictive analytics is both a powerful tool and a double-edged sword. Recognizing its limitations can pave the way for more responsible and beneficial applications of technology."
Understanding these challenges and limitations not only equips analysts but also assures investors and stakeholders that their interests are considered, fostering a responsible approach to predictive analytical models.
Future Trends in Predictive Analytics
As we look ahead, one cannot help but notice the pivotal role predictive analytics plays in shaping decision-making processes across a variety of sectors. The advent of new technologies and methodologies is steering the course of this field in impactful ways. Understanding these trends is crucial for stakeholders ranging from investors, educators, to analysts, as it opens the door to new possibilities.
Machine Learning and AI Integration
The integration of machine learning and artificial intelligence is reshaping the landscape of predictive analytics. These technologies enhance the accuracy and efficiency of predictive models, enabling them to sift through vast amounts of data. Leveraging algorithms that learn from patterns improves not only predictions but also allows for real-time adjustments based on new information. This adaptability is a game changer.
For instance, in the financial sector, AI-driven models can analyze market conditions, client behavior, and transaction patterns almost instantaneously. By utilizing tools like TensorFlow or PyTorch, financial analysts can develop models that are not only faster but also refine their predictive capabilities. Investors armed with these advanced tools can make informed decisions rather than relying on gut feelings or outdated data analyses.
Moreover, these integrations facilitate personalization, which is becoming a buzzword in customer experience. Businesses can tailor their services to meet individual customer needs by predicting future behavior based on previous interactions.
Real-Time Analytics
Another hot topic in predictive analytics is real-time analytics. The speed at which data is processed has increased dramatically over recent years. Real-time analytics allow organizations to react on-the-fly to changing conditions or new data, rather than working with stagnant datasets. This immediacy enhances overall responsiveness and can significantly drive competitive advantage.
For example, consider a retail company that tracks customer purchasing habits as they happen. By analyzing data in the moment, they might adjust their marketing strategies or inventory levels instantly. The ability to make changes while an event is unfolding demonstrates real operational excellence and a proactive stance that can influence profitability.
Keeping in mind, however, real-time analytics does come with its own set of challenges, such as the need for robust IT infrastructure and skilled personnel to interpret the data effectively.
Big Data and Predictive Models
Big data has emerged as a cornerstone of predictive analytics. With data being generated at unprecedented rates, harnessing this information has become both a necessity and a challenge. The convergence of various data points from multiple sources such as social media, IoT devices, and online transactions creates opportunities for predictive models to achieve greater accuracy.
Employing big data not only supports the refinement of predictive algorithms but also opens avenues for improved decision-making. Businesses can identify trends and insights that were previously obscured by smaller datasets. For instance, by combining internal sales data with external social trends, a company could accurately forecast product demands or potential market shifts.
Yet, it is essential to highlight that managing big data isn't just about accumulating vast amounts of information. It requires implementing efficient data management systems and frameworks that can process the data meaningfully.
The future of predictive analytics is intertwined with these technological innovations and paradigm shifts. Stakeholders must stay informed and agile to leverage these trends effectively, ensuring they are not left in the dust as the analytical landscape evolves.
"Adapting to trends in predictive analytics requires both foresight and flexibility. Those who can pivot are the ones who will thrive."
Ending
The conclusion of this article on predictive analytical models serves as the final corner-stone, wrapping up the diverse and intricate topics we've explored. Understanding the importance of predictive analytics is crucial for anyone engaged in data-driven decision-making processes. This section emphasizes several specific elements that resonate with not just the seasoned investor but also with educators, analysts, and students alike.
Recap of Key Insights
As we draw our findings to a close, it's vital to recap the core insights derived throughout the article. Here are the significant points that encapsulate our discussion:
- Definition and Historical Context: Predictive analytics is rooted in statistical methods that have evolved significantly over the last few decades. This evolution has led to sophisticated algorithms capable of discerning patterns from historical data.
- Model Development and Types: From regression to classification and time series models, we examined various methodologies suitable for addressing specific predictive tasks. Each model type offers unique benefits and tools for different scenarios.
- Evaluation Metrics: The necessity of thorough model assessment is unmistakable. Metrics such as accuracy, precision, recall, and ROC curves furnish critical insights that ensure optimal performance.
- Challenges in Practice: Issues such as data quality, overfitting, and ethical considerations present hurdles in deploying effective predictive models, necessitating ongoing vigilance and adaptation.
- Future Trends: The integration of machine learning and AI into predictive analytics paves the way for real-time insights, linking predictive models to vast data landscapes, thus broadening their application horizons.
Through these insights, it becomes evident that predictive analytics is not merely a tool but a transformative approach that can redefine how businesses strategize and operate.
Implications for Future Research
Looking ahead, the implications for future research are profound. As predictive analytics continues to permeate various sectors, several avenues warrant deeper exploration:
- Ethical Frameworks: One critical area is the development of ethical frameworks guiding the use of predictive models. The interplay between robust algorithmic predictions and ethical constraints must be navigated carefully, balancing innovation with responsibility.
- Real-Time Applications: As technology advances, a focus on real-time analytics will become more prominent. Research that explores the implications of real-time data processing on decision-making will be essential.
- Big Data Synergies: The synergy between big data technologies and predictive modeling is ripe for exploration. Further inquiry into how these relationships can enhance marketing strategies or operational efficiencies could yield significant benefits.
- Customization and Personalization: Tailoring predictive models to diverse audiences presents a path for personalized analytics that can drive customer satisfaction and enhance business outcomes.